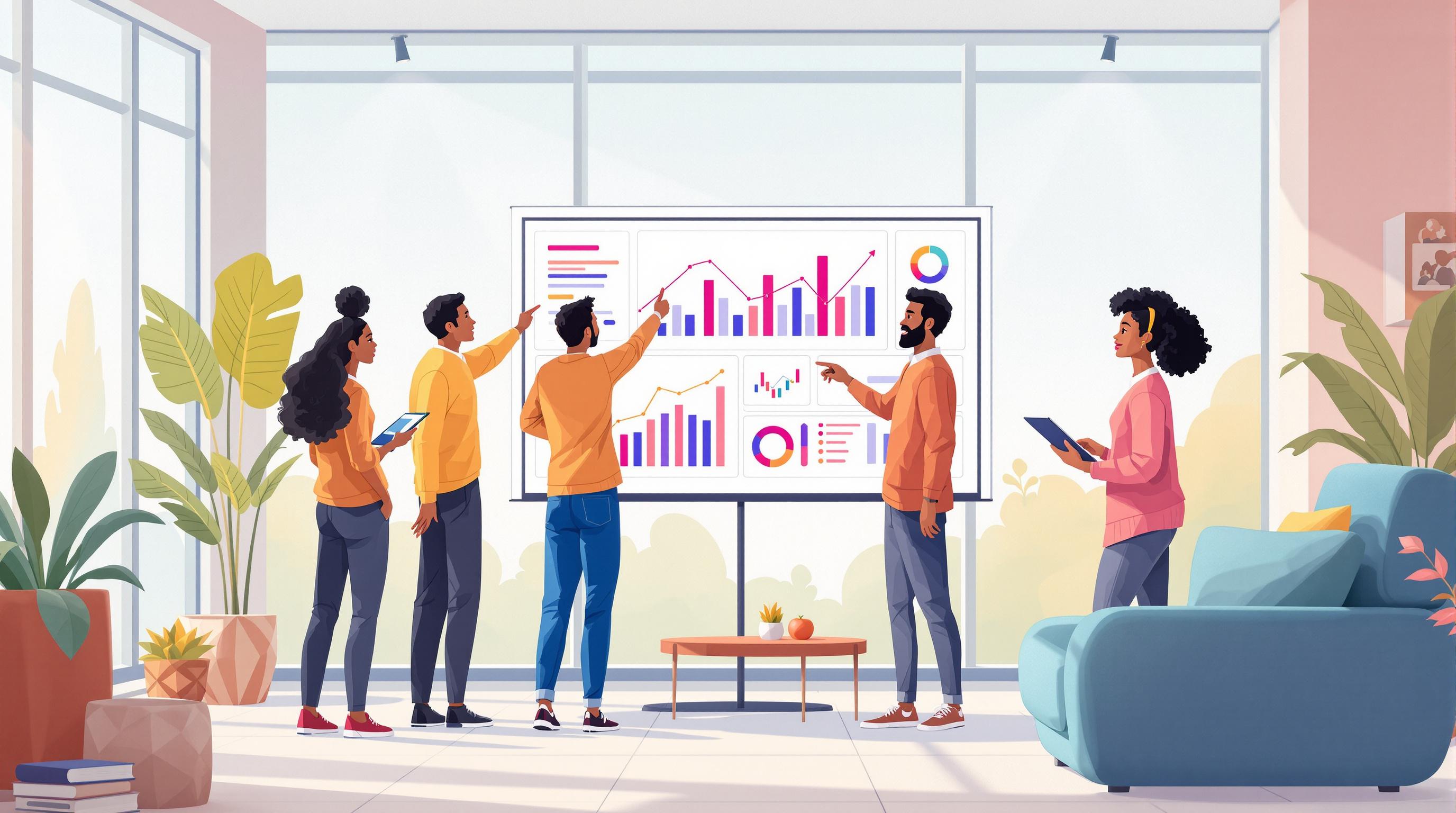
Low feature adoption is a common challenge for SaaS products, directly impacting user retention, satisfaction, and revenue. Here's how you can solve it using a data-driven approach:
Key Insights:
- Identify Barriers: Common issues include poor onboarding, low visibility, and usability problems.
- Analyze User Behavior: Tools like Screeb (session replays) and Amplitude (analytics) help uncover friction points and adoption trends.
- Combine Data with Feedback: Behavioral data shows what users do; feedback explains why they do it.
- Test and Optimize: Use A/B testing to refine onboarding, feature placement, and communication strategies.
Quick Steps to Improve Feature Adoption:
- Track Metrics: Measure feature awareness, activation, and usage frequency.
- Refine Onboarding: Personalize experiences for different user segments.
- Encourage Engagement: Use targeted, timely in-app messages.
- Iterate Continuously: Regularly analyze data and adjust features based on user needs.
By focusing on user behavior and feedback, you can systematically reduce friction, optimize features, and boost adoption rates.
Identifying the Reasons Behind Low Feature Adoption
Common Barriers: Onboarding, Awareness, and Usability
Low feature adoption often happens because of weak onboarding, lack of visibility, or usability problems. Users might not understand the feature's value, have trouble locating it, or stop using it due to frustrating experiences. Tools like Screeb's session replays can highlight where users run into these issues, such as steps with high drop-off rates or abandonment [1].
Barrier Type | Impact | How to Detect |
---|---|---|
Poor Onboarding | Users miss the feature's value | Analytics, drop-off rates |
Low Visibility | Users don't notice the feature | Heatmaps, discovery metrics |
Usability Problems | Users give up on the feature | Session replays, abandonment rates |
Fixing these barriers is important, but it’s equally important to ensure the feature solves real user problems.
When Features Don't Match User Needs
Even well-designed features can struggle if they don’t solve the right problems for users. This mismatch happens when features fail to address user pain points or expectations, causing users to lose interest [1]. By combining behavioral data with direct user feedback, teams can figure out why a feature isn’t connecting with users [3].
Signs of a mismatch between features and user needs include:
- Low engagement across different user groups
- Negative feedback in surveys or support tickets
- High abandonment rates after initial use [2]
Using Data to Understand Feature Engagement
Tools for Behavior Analysis: Screeb, Amplitude, and Others
Platforms like Screeb and Amplitude help teams uncover how users interact with features. Screeb uses AI to highlight patterns in how users discover features, while Amplitude focuses on tracking adoption trends across different user groups. Paired with visualization tools like heatmaps, these tools offer a detailed look at user behavior [1].
Analysis Tool | Key Capabilities | Primary Use Case |
---|---|---|
Screeb | Session replays, User journey analysis | Spotting friction points |
Amplitude | Feature usage tracking, Engagement metrics | Measuring adoption trends |
Heatmaps | Click patterns, Scroll depth | Visualizing feature discovery |
While these tools excel at providing numbers and patterns, combining them with user feedback paints a clearer picture of the challenges users face.
Combining User Feedback with Behavioral Data
Behavioral data tells you what users are doing, but feedback explains why they’re doing it [1]. By merging these two perspectives, teams can better understand the obstacles preventing users from fully adopting features.
To address these barriers systematically, the ARIA framework works as a guide alongside tools like Screeb and Amplitude:
- Analyze: Study both behavior data and feedback.
- Reduce: Eliminate friction points in the user journey.
- Introduce: Present features in a way that makes sense to users.
- Assist: Offer support to help users succeed [3].
Testing Features with A/B Experiments
A/B testing allows teams to test assumptions and refine features through controlled trials [1]. For instance, Wudpecker.io boosted feature adoption by 20% in just three months by experimenting with onboarding flows and tailoring in-app messages. Their tests focused on key elements like when to introduce features, where to place them in the UI, the onboarding process, and how easy it was for users to access help documentation [1].
"Feature adoption drives product success. Analyze, reduce friction, and optimize for engagement." - Statsig, 2024 [3]
Practical Steps to Improve Feature Adoption
Customizing Onboarding and In-App Help
Design onboarding experiences that cater to different user segments by introducing features gradually, based on their level of engagement and readiness. Present features at moments when users are most likely to find them helpful and relevant. This method avoids overwhelming users and increases the chances of them adopting the features successfully [1].
Once the onboarding process is tailored to user needs, reinforce feature adoption with timely and relevant communication.
Encouraging Use with Targeted Messages
Boost adoption by sending well-timed messages during key moments, such as feature discovery, activation, and post-use reinforcement. The timing and context of these prompts play a crucial role in encouraging users to explore and continue using features [1]. For instance, when users complete a specific workflow, suggest related features that naturally extend their experience within the product.
While these targeted messages help spark initial interest, ongoing adjustments based on user data are essential for maintaining engagement.
Refining Features Based on Data
To ensure features remain useful and engaging over time, use data to guide refinements. Track key metrics that highlight how features are performing:
Metric | Insight for Action |
---|---|
Feature Awareness | Make features easier to find |
Activation Rate | Simplify the first-use experience |
Usage Frequency | Focus on delivering consistent value |
Prioritize updates based on quantitative data, while also considering user feedback. This combination helps teams identify and address real user challenges rather than relying on assumptions [1].
"Feature adoption drives product success. Analyze, reduce friction, and optimize for engagement." - Statsig, 2024 [3]
sbb-itb-5851765
Driving Product Adoption using Behavioral Analysis
Case Study: Improving Feature Adoption with Data
Here’s a real-world example of how a SaaS company addressed low feature adoption using data-driven strategies.
Understanding the Problem and Initial Findings
A mid-sized SaaS company noticed that only 12% of users were adopting their new collaboration features after launch. By analyzing session replays and behavioral data, they uncovered key issues:
Issue | Data Source | Key Finding |
---|---|---|
Discovery | Session Replays | 67% of users never clicked the feature menu |
Onboarding | User Journey Analysis | Most users abandoned the setup process |
Interface | Behavioral Data | Tasks took 3x longer than expected |
These findings highlighted problems with how users discovered and interacted with the feature, as well as the setup process itself.
Solutions Applied and Results Achieved
The product team took a targeted approach to address these challenges:
- Improved Feature Discovery: Using heatmap data, they redesigned the interface to make collaboration features more visible. This boosted feature discovery by 45% within the first month.
- Simplified Onboarding: A/B testing showed that adding contextual tooltips and guides significantly reduced setup drop-off rates, cutting them by 51%.
- Optimized Interface: By analyzing user behavior, the team streamlined workflows and reduced task completion time by 65%. Automated in-app prompts were added to assist users at critical steps.
Lessons Learned and Tips for Success
These changes led to a sharp rise in feature adoption, providing valuable insights for tackling similar challenges. The team’s focus on integrating user feedback with data analysis proved crucial. Iterative testing ensured that each solution had a measurable impact.
Here’s how the key metrics improved over three months:
Metric | Initial | After 3 Months |
---|---|---|
Feature Discovery | 33% | 78% |
Setup Completion | 18% | 69% |
This case clearly demonstrates how a structured, data-driven approach can drive meaningful improvements in feature adoption when tracked and refined over time [1] [3].
Conclusion and Next Steps
Key Strategies Recap
Boosting feature adoption starts with understanding user behavior. The ARIA framework offers a clear method to identify and improve feature usage. Companies that excel in this area focus on these three main strategies:
Strategy Component | Key Activities | Impact Indicators |
---|---|---|
Behavioral Analysis | Session recordings, heatmaps, click analytics | Insights into how users interact with features |
User Segmentation | Personalized experiences, targeted messaging | Higher rates of feature discovery |
Iterative Testing | A/B testing, feature refinement | Noticeable improvements in adoption metrics |
The case study shows how applying these strategies over time can lead to better adoption rates [1] [3].
How to Begin
Improving feature adoption starts by measuring your baseline across four critical stages: Exposed, Activated, Used, and Used Again [2]. From there, take these steps:
-
Set Up Analytics Tools
Use platforms like Screeb or Amplitude to track user behavior and gather feedback [1]. -
Define Your Metrics
Focus on metrics that provide meaningful insights, such as: -
Commit to Continuous Improvement
Regularly review your metrics, tweak features monthly, and evaluate strategies every quarter [1] [3].
By following these steps, you can integrate these strategies into your product development process. Start with clear goals, rely on data to guide decisions, and keep refining based on how users interact with your product [1].
"Feature adoption drives product success. Analyze, reduce friction, and optimize for engagement." - Statsig, 2024 [3]
FAQs
What is feature usage in analytics?
Feature usage tracks how many users interact with specific features in your product. It’s calculated using this formula:
(Users Using Feature / Total Product Users) × 100.
For example, if 2,500 out of 10,000 users use a feature, the feature usage rate is 25%.
Metric | Description | Why It Matters |
---|---|---|
Time to First Use | Time between signup and first feature use | Helps gauge the success of onboarding |
Usage Frequency | How often users interact with the feature | Highlights how engaging the feature is |
Feature Abandonment | Percentage of users who stop using a feature | Pinpoints potential usability issues |
Breaking down feature usage by user segments often uncovers key insights. For instance, enterprise users might adopt advanced features at a rate of 45%, while small business users lag behind at 15%. These patterns help identify where to focus improvement efforts [1][3].